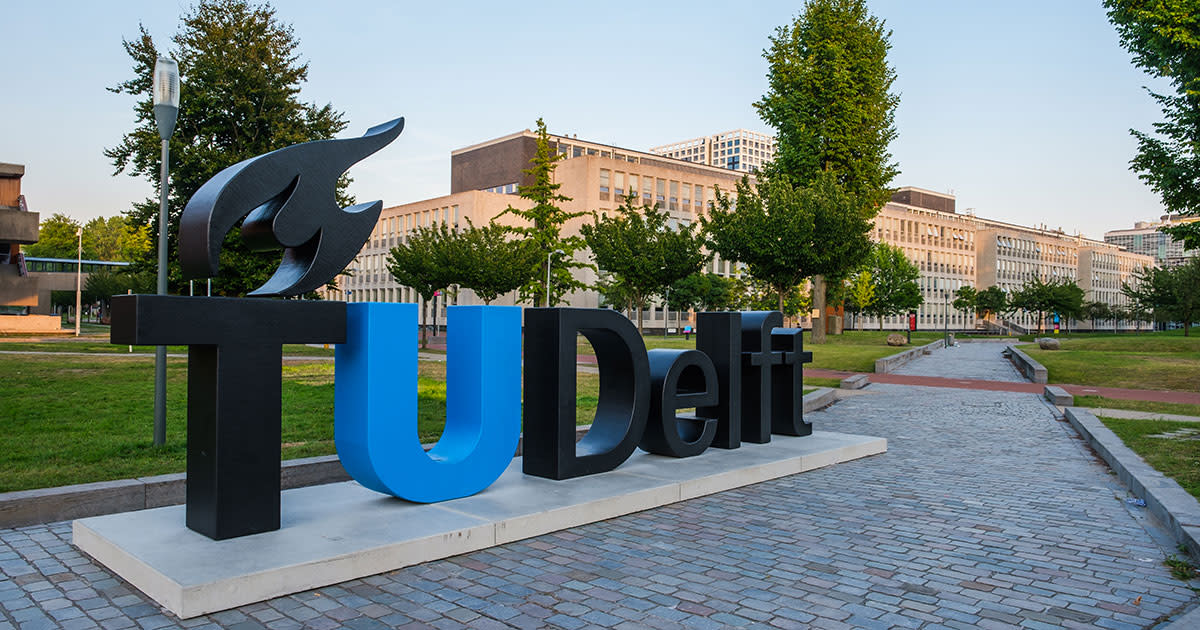
Access a .pdf version of the technical program and the program-at-a-glance here.
Program-At-A-Glance
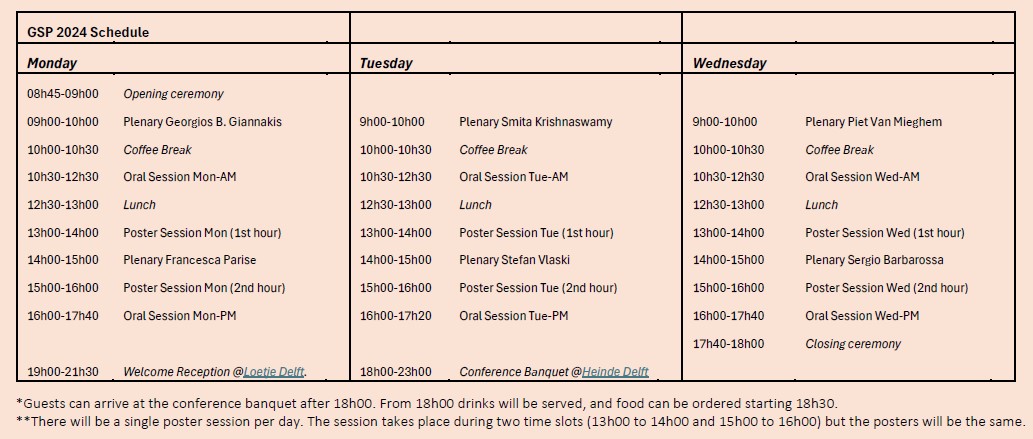
General Information
Lectures in the oral sessions are 20-minutes long (including Q&As). The format of posters is flexible, but A0 size and portrait orientation are recommended.
There will be a single poster session per day. The session takes place during two different time slots (13h00 to 14h00 and 15h00 to 16h00) but the posters will be the same.
There will be two social events:
- Welcome Reception: Monday 24 June, 19:00-21:30 @Loetje Delft
- Conference Banquet: Tuesday 25 June, 18:00-23:00 @Heinde Delft
Plenary Talks
Plenary Monday 9:00 - 10:00
Georgios B. Giannakis, University of Minnesota
Title: Kernel-driven and Learnable Self-Supervision over Graphs
Abstract: Self-supervision (SeSu) has gained popularity for data-hungry training of machine learning models, especially those involving large-scale graphs, where labeled samples are scarce or even unavailable. Main learning tasks in such setups are ill posed, and SeSu renders them well posed by relying on abundant unlabeled data as input, to yield low-dimensional embeddings of a reference (auxiliary) model output. In this talk, we first outline SeSu approaches, specialized reference models, and their links with (variational) auto-encoders, regularization, semi-supervised, transfer, meta, and multi-view learning; but also their challenges and opportunities when multi-layer graph topologies and multi-view data are present, when nodal features are absent, and when the ad hoc selection of a reference model yields embeddings not optimally designed for the downstream main learning task. Next, we present our novel SeSu approach which selects the reference model to output either a prescribed kernel, or, a learnable weighted superposition of kernels from a prescribed dictionary. As a result, the learned embeddings offer a novel, reduced-dimensionality estimate of the basis kernel, and thus an efficient parametric estimate of the main learning function at hand that belongs to a reproducing kernel Hilbert space. If time allows, we will also cover online variants for dynamic settings, and regret analysis founded on the so-termed neural-tangent-kernel framework to assess how effectively the learned embeddings approximate the underlying optimal kernel(s). We will wrap up with numerical tests using synthetic and real datasets to showcase the merits of kernel-driven and learnable (KeLe) SeSu relative to alternatives. The real data will also compare KeLe-SeSu with auto-encoders and graph neural networks (GNNs), and further test KeLe-Su on reference maps with masked-inputs and predicted-outputs that are popular in large language models (LLMs).
Plenary Monday 14:00 - 15:00
Francesca Parise, Cornell University
Title: Large-Scale Network Dynamics: Achieving Tractability via Graph Limits
Abstract: Network dynamical systems provide a versatile framework for studying the interplay between the structure of complex networks and the dynamic behavior of its constituent entities, offering insights into diverse phenomena across disciplines such as physics, biology, sociology, and engineering. As the size of the underlying network increases however a number of new challenges arise. For example, collecting exact network data may become too costly and planning optimal network interventions may become computationally intractable. In this talk I will show how the theory of graph limits can be used to provide new insights on graph processes evolving over large random networks. First, I will show how graph limits can be used to define tractable infinite population models of network systems while maintaining agents heterogeneity. Second, I will show how insights derived for such infinite population models can be applied to study large but finite networks. I will illustrate the benefit of this graph limit approach for broad classes of graph processes including strategic interactions, multi-agent learning and synchronization dynamics.
Plenary Tuesday 9:00 - 10:00
Smita Krishnaswamy, Yale University
Title: Molecular discovery and analysis using geometric scattering and deep learning
Abstract: Here we present frameworks that integrate graph signal processing with deep learning in order to enhance graph representation-learning as well as predictive modeling of graph dynamics. A key innovation is our development and incorporation of learnable geometric scattering as well as its novel extensions bilipschitz scattering, directed scattering and scattering with attention, as layers into deep neural networks in order to improve expressiveness and downstream performance. We first discuss enhanced expressiveness and capability of capturing geometric properties of these methods. Then, we show applications in molecule generation, dynamics interpolation, as well as property prediction in small molecules and proteins. Finally, I will show applications where the structure captured by the graph signal processing is augmented by sequence analysis to elucidate basic RNA transcriptional biology.
Plenary Tuesday 14:00 - 15:00
Stefan Vlaski, Imperial College London
Title: Beyond Consensus-Based Methods for Decentralized Learning: Where Graph Signal Processing and Optimization Meet
Abstract: Recent years have been marked by a proliferation of dispersed data and computational capabilities. Data is generated and processed on our mobile devices, in sensors scattered throughout smart cities and smart grids , and vehicles on the road. Central aggregation of raw data is frequently neither efficient nor feasible, due to concerns around communication constraints, privacy, and robustness to link and node failure. The purpose of decentralized optimization and learning is then to devise intelligent systems by means of decentralized processing and peer-to-peer interactions, as defined by an underlying graph topology. Classically, the objective in decentralized learning has been to match the dynamics and performance of a centralized fusion center, and minimize the impact of the network on the learning dynamics. In convex, cooperative, and homogeneous environments, this paradigm is indeed optimal and can be shown to match statistical and information theoretic lower bounds. Modern learning applications, however, differ substantially from classical settings: They are highly non-convex, underdetermined, heterogeneous, and subject to non-cooperative and adversarial behavior. We will argue that effective learning in these environments requires deviation from the traditional consensus-based learning paradigm, and a refined characterization of the inductive bias and learning dynamics induced by the underlying graph topology. We will survey recent results emerging from this point of view.
Plenary Wednesday 9:00 - 10:00
Piet Van Mieghem, TU Delft
Title: Linear Processes on Networks
Abstract: From a network science point of view, we will discuss linear processes on a graph, which are the easiest, but also the most elegant processes. Real, time-dependent processes necessitate us to introduce the beautiful linear state space (LSS) model (in discrete time). We will briefly talk about two applications of LSS. Thereafter, we switch to the Laplacian matrix of a graph. From the spectral decomposition of the Laplacian, we will introduce the simplex of an undirected, possibly weighted graph. The simplex geometry of a graph is, besides the topology domain and the spectral domain, the third equivalent description of a graph. Continuous-time Markov processes are described by the Chapman-Kolmogorov (linear) equations, in which the appearing infinitesimal generator is, in fact, a weighted Laplacian of the Markov graph. We will discuss Markovian epidemics on a fixed graph, show limitations of the linear theory on graphs and introduce a mean-field approximation, a powerful method from physics, that results into non-linear governing equations.
Plenary Wednesday 14:00 - 15:00
Sergio Barbarossa, Sapienza University of Rome
Title: Topological signal processing and learning over cell complexes
Abstract: The goal of this lecture is to introduce the basic tools for processing signals defined over a topological space, focusing on simplicial and cell complexes. Nowadays, processing signals defined over graphs has become a mature technology. Graphs are just an example of topological space, incorporating only pairwise relations. In this lecture, we will motivate the need to generalize the graph-based methodologies to higher order structures, such as simplicial and cell complexes as spaces able to incorporate higher order relations in the representation space, still possessing a rich algebraic structure that facilitates the extraction of information. We will motivate the introduction of a Fourier Transform and recall the fundamentals of filtering and sampling of signals defined over such spaces. We will then show the impact of imperfect knowledge of the space on the tools used to extract information from data. Then we will present a probabilistic topological model for multivariate random variables defined over subsets of the space. Finally, we will show how to exploit the above tools in the design of topological neural networks, operating over signals defined over topological spaces of different order and we will present methods to infer the structure of the space from data.
Oral Sessions
Oral Session Mon-AM (Monday 10:30 - 12:30) - Network Topology Inference
- Polynomial graphical lasso and generalizations. A. G. Marques (Rey Juan Carlos University), A. Buciulea (Rey Juan Carlos University), J. Ying (The Hong Kong University of Science and Technology), and D.P. Palomar (The Hong Kong University of Science and Technology).
- Mitigating subpopulation bias for fair network topology inference. Madeline Navarro (Rice University), Samuel Rey (Rey Juan Carlos University), Andrei Buciulea Vlas (Universidad Rey Juan Carlos), Antonio G. Marques (Rey Juan Carlos University), Santiago Segarra (Rice University).
- Inferring the Topology of a Networked Dynamical Systems. Augusto A Santos (Instituto de Telecomunica es), Jos M. F. Moura (Carnegie Mellon University).
- Sampling and Consensus for Anomalous Edge Detection. Abdullah Karaaslanli (Michigan State University), Panagiotis Traganitis (Michigan State University), Selin Aviyente (Michigan State University).
- Introducing Graph Learning over Polytopic Uncertain Graph. Masako Kishida (National Institute of Informatics), Shunsuke Ono (Tokyo Institute of Technology).
- Laplacian-Constrained Cramer-Rao Bound for Networks Applications. Morad Halihal (Ben-Gurion University of the Negev), Tirza S Routtenberg (Ben Gurion University of the Negev).
Oral Session Mon-PM (Monday 16:00 - 17:40) - Graph filters
- Involution-Based Graph-Signal Processing. Gerald Matz (Technische Universit t Wien), Dimitrios Kalodikis (TU Wien).
- On the Stability of Graph Spectral Filters: A Probabilistic Perspective. Ning Zhang (University of Oxford), Henry Kenlay (University of Oxford), Mihai Cucuringu (University of Oxford and The Alan Turing Institute), Xiaowen Dong (University of Oxford).
- Algebraic spaces of filters for signals on graphons. Alejandro Parada-Mayorga (University of Pennsylvania), Leopoldo Agorio (University of Pittsburgh), Alejandro Ribeiro (University of Pennsylvania), Juan Andres Bazerque (Univerity of Pittsburgh).
- Graph Filtering for Clustering Attributed Graphs. Meiby Ortiz-Bouza (Michigan State University), Selin Aviyente (Michigan State University).
- HoloNets: Spectral Convolutions do extend to Directed Graphs. Christian Koke (Technical University Munich), Daniel Cremers (TU Munich).
Oral Session Tue-AM (Tuesday 10:30 - 12:30) - Signal processing on higher-order networks
- Disentangling the Spectral Properties of the Hodge Laplacian: Not All Small Eigenvalues Are Equal. Vincent P. Grande (RWTH Aachen University), Michael Schaub (RWTH Aachen University).
- Simplicial Vector Autoregressive Models. Joshin P. Krishnan (Simula Metropolitan Center for Digital Engineering), Rohan Thekkemarickal Money (Simula), Baltasar Beferull-Lozano (Simula Metropolitan Center for Digital Engineering), Elvin Isufi (Tu Delft).
- Learning Graphs and Simplicial Complexes from Data. Andrei Buciulea Vlas (Rey Juan Carlos University), Elvin Isufi (Tu Delft), Geert Leus (TU Delft), Antonio G. Marques (Rey Juan Carlos University).
- Topological Dictionary Learning. Claudio Battiloro (Sapienza University of Rome), Paolo Di Lorenzo (Sapienza University of Rome), Alejandro Ribeiro (University of Pennsylvania).
- Hyperedge Representations with Deep Hypergraph Wavelets: Applications to Spatial Transcriptomics. Xingzhi Sun (Yale University), Charles Xu (Yale University), Benjamin Hollander-Bodie (Yale University), Laney Goldman (Harvey Mudd College), Michael Perlmutter (University of California, Los Angeles), Marcello DiStasio (Yale University), Smita Krishnaswamy (Yale University).
- Graph Neural Networks for predicting Chemical Toxicity, Akhil Gopinath (MathWorks).
Oral Session Tue-PM (Tuesday 16:00 - 17:20) - Graph learning
- Graph Topology Learning with Functional Priors. Chenyue Zhang (The Chinese University of Hong Kong), Hoi-To Wai (Chinese University of Hong Kong), Shangyuan LIU (The Chinese University of Hong Kong).
- Enhanced Graph-Learning Schemes Driven by Similar Distributions of Motifs. Samuel Rey (Rey Juan Carlos University), T. Mitchell Roddenberry (Rice University), Santiago Segarra (Rice University), Antonio G. Marques (Rey Juan Carlos University).
- Heterogeneous Graph Structure Learning: A Statistical Perspective. Keyue Jiang (University College London), Bohan Tang (University of Oxford), Laura Toni (UCL), Xiaowen Dong (University of Oxford).
- Graph Structure Learning with Interpretable Bayesian Neural Networks. Max Wasserman (University of Rochester), Gonzalo Mateos (University of Rochester).
Oral Session Wed-AM (Wednesday 10:30 - 12:30) - Geometric deep learning
- Online Time Covariance Neural Networks. Andrea Cavallo (Delft University of Technology), Mohammad Sabbaqi (Delft University of Technology), Elvin Isufi (Tu Delft).
- From Latent Graph to Latent Topology Inference: Differentiable Cell Complex Module. Claudio Battiloro (Sapienza University of Rome), Indro Spinelli (Sapienza University of Rome), Lev Telyatnikov (Sapienza University), Michael Bronstein (Oxford University), Simone Scardapane (Sapienza University), Paolo Di Lorenzo (Sapienza University of Rome).
- Graph Convolutional Neural Networks in the Companion Model. John Shi (Carnegie Mellon University), Shreyas Chaudhari (Carnegie Mellon University), Jos M. F. Moura (Carnegie Mellon University).
- Representing Edge Flows on Graphs via Sparse Cell Complexes. Josef Hoppe (RWTH Aachen University), Michael Schaub (RWTH Aachen University).
- Multi-Scale Hydraulic Graph Neural Networks. Roberto Bentivoglio (TU Delft), Elvin Isufi (Tu Delft), Sebastiaan Nicolas Jonkman (TU Delft), Riccardo Taormina (TU Delft).
- Simplicial Scattering Networks. Hiren Madhu (Indian Institute of Science, Bengaluru), Sravanthi Gurugubelli (Indian Institute of Science), Sundeep Prabhakar Chepuri (Indian Institute of Science).
Oral Session Wed-PM (Wednesday 16:00 - 17:40) - GSP Theory and Methods
- Quantile-based fitting for graph signals. Kyusoon Kim (Seoul National University).
- Mobius Total Variation for Directed Acyclic Graph. Vedran Mihal (ETH Zurich), Markus P schel (ETH Zurich).
- Median Autoregressive Graph Filters. David Tay (Deakin University).
- Blind Deconvolution of Sparse Graph Signals in the Presence of Perturbations. Victor M. Tenorio (Rey Juan Carlos University), Samuel Rey (Rey Juan Carlos University), Antonio G. Marques (Rey Juan Carlos University).
- Discrete Integral Operators for Graph Signal Processing. Naoki Saito (University of California, Davis), Eugene Shvarts (University of California, Davis).
Poster Sessions
Poster Session Mon (Monday 13:00-14h00 & 15h00-16h00) - Euler
- Decentralized and Lifelong-Adaptive Multi-Agent Collaborative Learning. Shuo Tang (Stanford University), Rui Ye (Shanghai Jiao Tong University), Chenxin Xu (Shanghai Jiao Tong University), Xiaowen Dong (University of Oxford), Siheng Chen (Shanghai Jiao Tong University, Shanghai AI Laboratory), Yan-Feng Wang (Cooperative medianet innovation center of Shanghai Jiao Tong University).
- Online Graph Learning Via Proximal Newton Method from Streaming Data. Zu-Yu Wu (National Yang Ming Chiao Tung University), Carrson C. Fung (Carnegie Mellon University), Jun-Yi Chang (National Yang Ming Chiao Tung University), Hsin Chuang (National Yang Ming Chiao Tung University), Yi-Chen Lin (National Yang Ming Chiao Tung University).
- Online Graph Filtering Over Expanding Graphs. Bishwadeep Das (TU Delft), Elvin Isufi (Tu Delft)
- Hodge-Aware Matched Subspace Detectors. Chengen Liu (Tu Delft), Elvin Isufi (Tu Delft).
- Windowed Hypergraph Fourier Transform and Vertex-frequency Representation. Alcebiades Dal Col (Federal University of Espirito Santo), Fabiano Petronetto (Federal University of Espirito Santo), Juliano B. Lima (Federal University of Pernambuco), Jos R de Oliveira Neto (Universidade Federal de Pernambuco).
- Graph Signal Processing: The 2D Companion Model. John Shi (University of Michigan), Jos M. F. Moura (Carnegie Mellon University).
- Robust Graph Learning for Classification. Jingxin Zhang (Swinburne University of Technology), Subbareddy BATREDDY (IIT HYDERABAD), Aditya Siripuram (IIT Hyderabad).
- Sparse Recovery of Diffused Graph Signals. Gal Morgenstern (Ben-Gurion University of the Negev), Tirza S. Routtenberg (Ben-Gurion University of the Negev).
- Learning Stochastic Graph Neural Networks with Constrained Variance. Zhan Gao (University of Cambridge), Elvin Isufi (Tu Delft).
- On the Impact of Sample Size in Reconstructing Signals with Graph Laplacian Regularisation. Baskaran Sripathmanathan (University of Oxford), Xiaowen Dong (University of Oxford), Michael Bronstein (University of Oxford).
- Hypergraph Transformer for Semi-Supervised Classification. Zexi Liu (Shanghai Jiao Tong University), Bohan Tang (University of Oxford), Ziyuan Ye (The Hong Kong Polytechnic University), Xiaowen Dong (University of Oxford), Yanfeng Wang (Shanghai Jiao Tong University), Siheng Chen (Shanghai Jiao Tong University, Shanghai AI Laboratory).
- Convolutional GNN to process signals defined over DAGs. Samuel Rey (Rey Juan Carlos University), Hamed Ajorlou (University of Rochester), Gonzalo Mateos (University of Rochester).
- Graph Neural Networks with Adaptive Structure. Zepeng Zhang (EPFL), Songtao Lu (IBM Thomas J. Watson Research Center), Zengfeng Huang (Fudan University), Ziping Zhao (ShanghaiTech University).
- Efficient Task Planning with Taxonomy Graph States and Large Language Models. Rodrigo P rez Dattari (TU Delft), Zhaoting Li (TU Delft), Robert Babuska (TU Delft), Jens Kober (TU Delft), Cosimo Della Santina (TU Delft).
- Sampling sparse graph signals. Stefan Kunis (University of Osnabrueck).
- Learned Finite-Time Consensus for Distributed Optimization. Aaron Fainman (Imperial College London), Stefan Vlaski (Imperial College London).
Poster Session Tue (Tuesday 13:00-14h00 & 15h00-16h00) - Laplace
- ResolvNet: A Graph Convolutional Network with multi-scale Consistency. Christian Koke (TU Munich), Abhishek Saroha (TU Munich), Yuesong Shen (TU Munich), Marvin Eisenberger (TU Munich), Daniel Cremers (TU Munich).
- Multiscale Graph Signal Clustering. Reina Kaneko (Tohoku University), Kenta Yanagiya (Osaka University), Junya Hara (Osaka University), Hiroshi Higashi (Osaka University), Yuichi Tanaka (Osaka University).
- On Stability of GCNN Under Graph Perturbations. Jun Zhang (ShanghaiTech University), Ziping Zhao (ShanghaiTech University).
- Kernel graph filtering – a new method for dynamic sinogram denoising. Jingxin Zhang (Swinburne University of Technology), Shiyao Guo (GuiZhou University of Finance and Economics).
- Data-Aware Dynamic Network Decomposition. Bishwadeep Das (TU Delft), Andrei Buciulea Vlas (Rey Juan Carlos University), Antonio G. Marques (Rey Juan Carlos University), Elvin Isufi (Tu Delft).
- Hodge-Compositional Edge Gaussian Processes. Maosheng Yang (TU Delft), Viacheslav Borovitskiy (ETH Z rich), Elvin Isufi (Tu Delft).
- Estimators for Connection-Laplacian-Based Linear Algebra. Hugo Jaquard (GIPSA-lab), Nicolas Tremblay (CNRS), Simon Barthelm (CNRS), Pierre-Olivier Amblard (CNRS).
- Recovering Missing Node Features with Local Structure-based Embeddings. Victor M. Tenorio (Rey Juan Carlos University), Madeline Navarro (Rice University), Santiago Segarra (Rice University) Antonio G. Marques (Rey Juan Carlos University).
- Seeking universal approximation for continuous counterparts of GNNs on large random graphs. Matthieu Cordonnier (GIPSA-lab), Nicolas Tremblay (CNRS, GIPSA-lab), Nicolas Keriven (CNRS, GIPSA-lab), Samuel Vaiter (CNRS, Lab. J.A Dieudonn ).
- Benchmarking Graph Neural Networks with the Quadratic Assignment Problem. Adrien Lagesse (INRIA, ENS Paris), Marc Lelarge (INRIA-ENS).
- Inferring Time-Varying Signal over Uncertain Graphs. Mohammad Sabbaqi (TU Delft).
- Optimal Quasi-clique: Hardness Equivalence with Densest-k-Subgraph, and Quasi-partitioned Community Mining. Aritra Konar (KU Leuven), Nicholas D. Sidiropoulos (University of Virginia).
- Learning Causal Influences from Social Interactions. Mert Kayaalp (EPFL), Ali H. Sayed (EPFL).
- Peer-to-Peer Learning + Consensus with Non-IID Data. Srinivasa Pranav (Carnegie Mellon University), Jos M. F. Moura (Carnegie Mellon University
- Community mining by modeling multilayer networks with Cartesian product graphs. Tiziana Cattai (Sapienza University of Rome), Stefania Colonnese (Sapienza University of Rome, Italy).
- A Rewiring Contrastive Patch PerformerMixer Framework for Graph Representation Learning. Zhongtian Sun (Durham University), Anoushka Harit (Durham University), Alexandra Cristea (Durham University), Jingyun Wang (Durham university), Pietro Li (University of Cambridge), Jongmin Yu (University of Cambridge).
- Blind identification of overlapping communities from nodal observations. Ruben Wijnands (TU Delft), Geert Leus (TU Delft), Borbala Hunyadi (TUDelft).
- GSP-Traffic Dataset: Graph Signal Processing Dataset Based on Traffic Simulation. Rui Kumagai (Osaka University), Hayate KOJIMA (Tokyo University of Agriculture and Technology), Hiroshi Higashi (Osaka University), Yuichi Tanaka (Osaka University).
- A Novel Smoothness Prior for Hypergraph Machine Learning. Bohan Tang (University of Oxford), Siheng Chen (Shanghai Jiao Tong University, Shanghai AI Laboratory), Xiaowen Dong (University of Oxford).
Poster Session 3 (Wednesday 13:00-14h00 & 15h00-16h00) - Erdos
- Utilizing graph Fourier transform for automatic Alzheimer s disease detection from EEG signals. Ramnivas Sharma (MNIT Jaipur), Hemant Kumar Meena (MNIT Jaipur).
- Emergence of Higher-Order Functional Brain Connectivity with Hypergraph Signal Processing. Breno C. Bispo (University of Amsterdam), Fernando Santos (University of Amsterdam), Jos R de Oliveira Neto (Universidade Federal de Pernambuco), Juliano B Lima (Federal University of Pernambuco).
- Protection Against Graph-Based False Data Injection Attacks on Power Systems. Gal Morgenstern (Ben-Gurion University of the Negev), Tirza S Routtenberg (Ben Gurion University of the Negev), Gil Zussman (Columbia University), James Anderson (Columbia University), Jip Kim (Korea Institute of Energy Technology).
- Arbitrarily Sampled Signal Reconstruction Using Relative Difference Features. Chin-Yun Yu (Queen Mary University of London), Johan Pauwels (Queen Mary University of London), George Fazekas (QMUL).
- Incorporating the spiral of silence into opinion dynamics. Shir Mamia (Bar Ilan University).
- scPrisma infers, filters and enhances topological signals in single-cell data using spectral template matching. Jonathan Karin (The Hebrew University), Yonathan Bornfeld (The Hebrew University), Mor Nitzan (The Hebrew University).
- Graph Neural Network-Based Node Deployment for Throughput Enhancement. Yifei Yang (Wuhan University), Dongmian Zou (Duke Kunshan University), Xiaofan He (Wuhan University).
- Attack Graph Model for Cyber-Physical Power Systems Using Hybrid Deep Learning. Alfan Presekal (TU Delft).
- Interpretable Diagnosis of Schizophrenia Using Graph-Based Brain Network Information Bottleneck. Tianzheng Hu (Vrije University), Shujian Yu (VU Amsterdam).
- Exploiting Variational Inequalities for Generalized Change Detection on Graphs. Juan F. Florez (University of Delaware).
- Measuring Structure-Function Coupling Using Joint-modes of Multimodal Brain Networks. Sanjay Ghosh (Indian Institute of Technology Kharagpur), Ashish Raj (UCSF), Srikantan Nagarajan (UCSF).
- Autoregressive GNN for emulating Stormwater Drainage Flows. Alexander Garz n (TU Delft), Zoran Kapelan (TU Delft), Jeroen Langeveld (TU Delft / Partners4UrbanWater), Riccardo Taormina (TU Delft).
- Faster Convergence with Less Communication: Broadcast-Based Subgraph Sampling for Decentralized Learning over Wireless Networks. Daniel P rez Herrera (Link ping University), Zheng Chen (Link ping University), Erik G. Larsson (Nil).
- Data-driven Polytopic Output Synchronization from Noisy Data. Wenjie Liu (Beijing Institute of Technology), Yifei Li (Beijing Institute of Technology), Gang Wang (Beijing Institute of Technology).
- A Graph Signal Processing Framework based on Graph Learning and Graph Neural Networks for Mental Workload Classification from EEG signals. Maria a Sarkis (LS2N), Mira Rizkallah (Ecole Centrale Nantes), Sa d Moussaoui (LS2N - SIMS).
- State Estimation in Water Distribution Systems using Diffusion on the Edge Space. Bulat Kerimov (NTNU), Maosheng Yang (TU Delft), Riccardo Taormina (TU Delft), Franz Tscheikner-Gratl (NTNU).
- Neuro-GSTH: Quantitative analysis of spatiotemporal neural dynamics using geometric scattering and persistent homology. Dhananjay Bhaskar (Yale University), Jessica Moore (Duke University), Yanlei Zhang (Mila), Rahul Singh (Yale University), Valentina Greco (Yale University), Joy Hirsch (Yale University), Smita Krishnaswamy (Yale University).
- PET Image Representation and Reconstruction based on Graph Filter. Jingxin Zhang (Swinburne University of Technology), Shiyao Guo (GuiZhou University of Finance and Economics).